Categories
Recent Posts
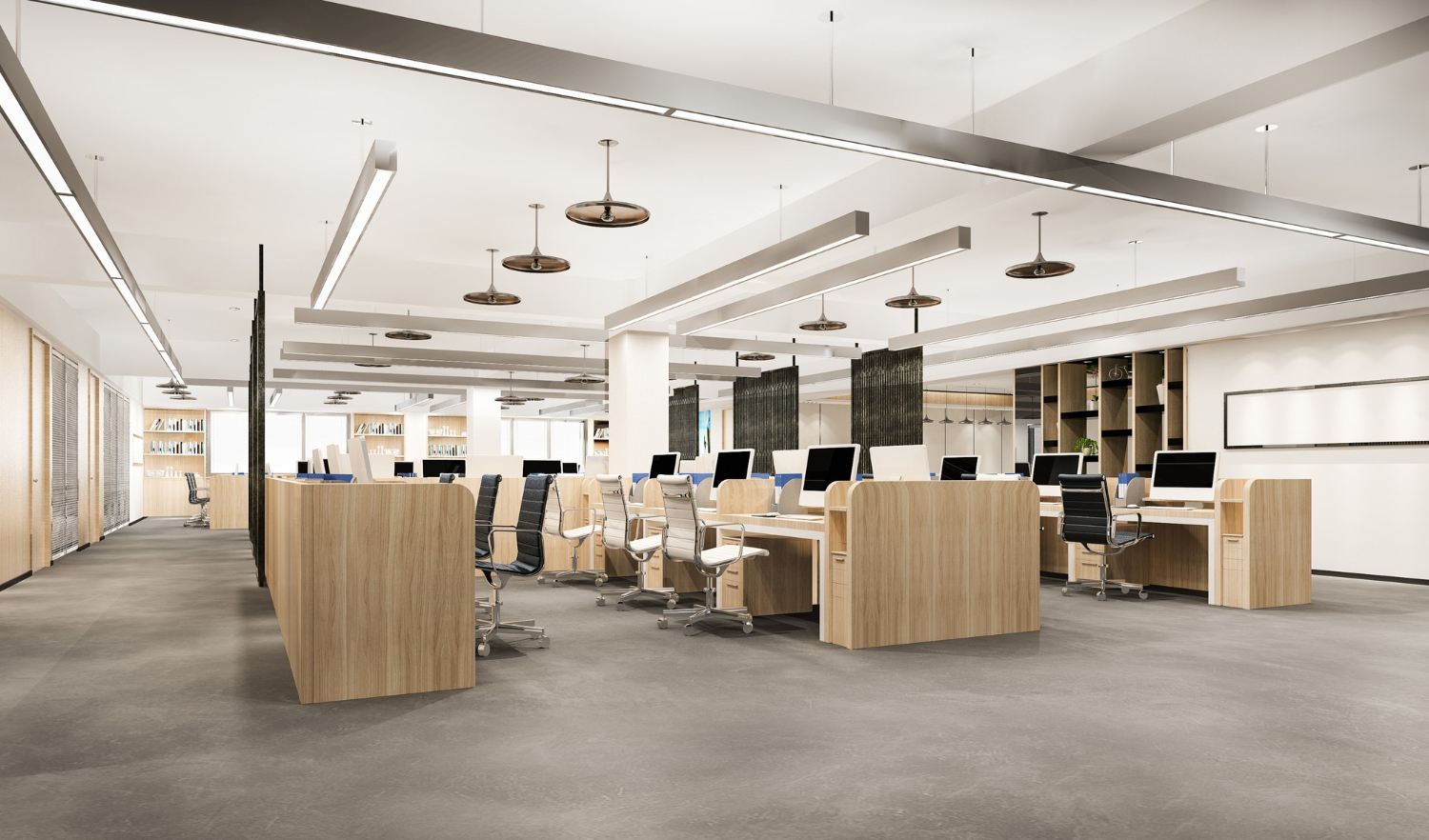
การมีทำเลที่ดีตามหลักสากลและหลักฮวงจุ้ย ย่อมดีกว่าทำเลไม่ดีเสมอ..ไม่ว่าจะเป็นในเรื่องของการเดินทาง พื้นที่จอดรถ สภาพภูมิประเทศ การจราจร การติดต่องานหรือแม้กระทั้งการหาของกิน ก็ล้วนแล้วแต่เป็นปัจจัยที่มีผลต่อการเลือกพื้นที่ตั้งเป็นสำนักทั้งสิ้นครับ บทความนี้จะขอพาทุกๆ ท่านไปพบกับ “5…
สิ่งที่ควรพิจารณาก่อนเลือกพื้นที่ตั้งเป็นสำนักงาน
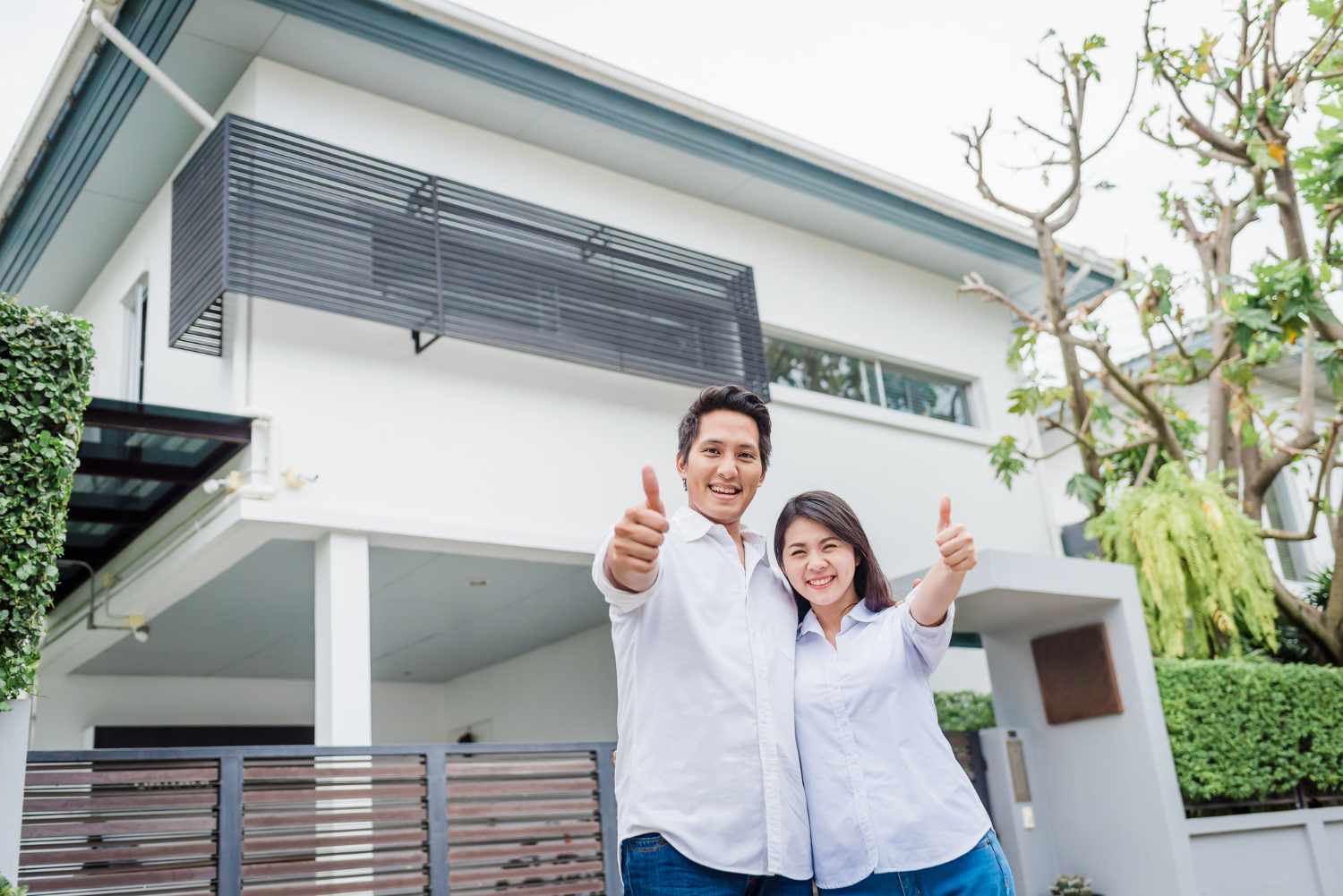
บ้าน…เป็นสิ่งที่ใครทุกคนใฝ่ฝันที่จะมีเป็นของตนเองสักหลัง เพราะบ้านจะเป็นทุกๆ อย่างให้กับเราไม่ว่าจะเป็นที่หลบภัย ที่พักผ่อนกายและฟื้นฟูจิตใจของเราทุกๆ คน ซึ่งบ้านที่ทุกๆ คนชอบก็จะแตกต่างกันออกไป แต่มีหลายสิ่งหลักๆ ที่คนที่กำลังจะมองหาบ้านเป็นของตนเองต้องคำนึงถึงเพื่อความเป็นอยู่ในอนาคต บทความนี้จะขอพาทุกๆ…
5 สิ่งที่ควรคำนึงถึงเมื่อต้องซื้อบ้าน
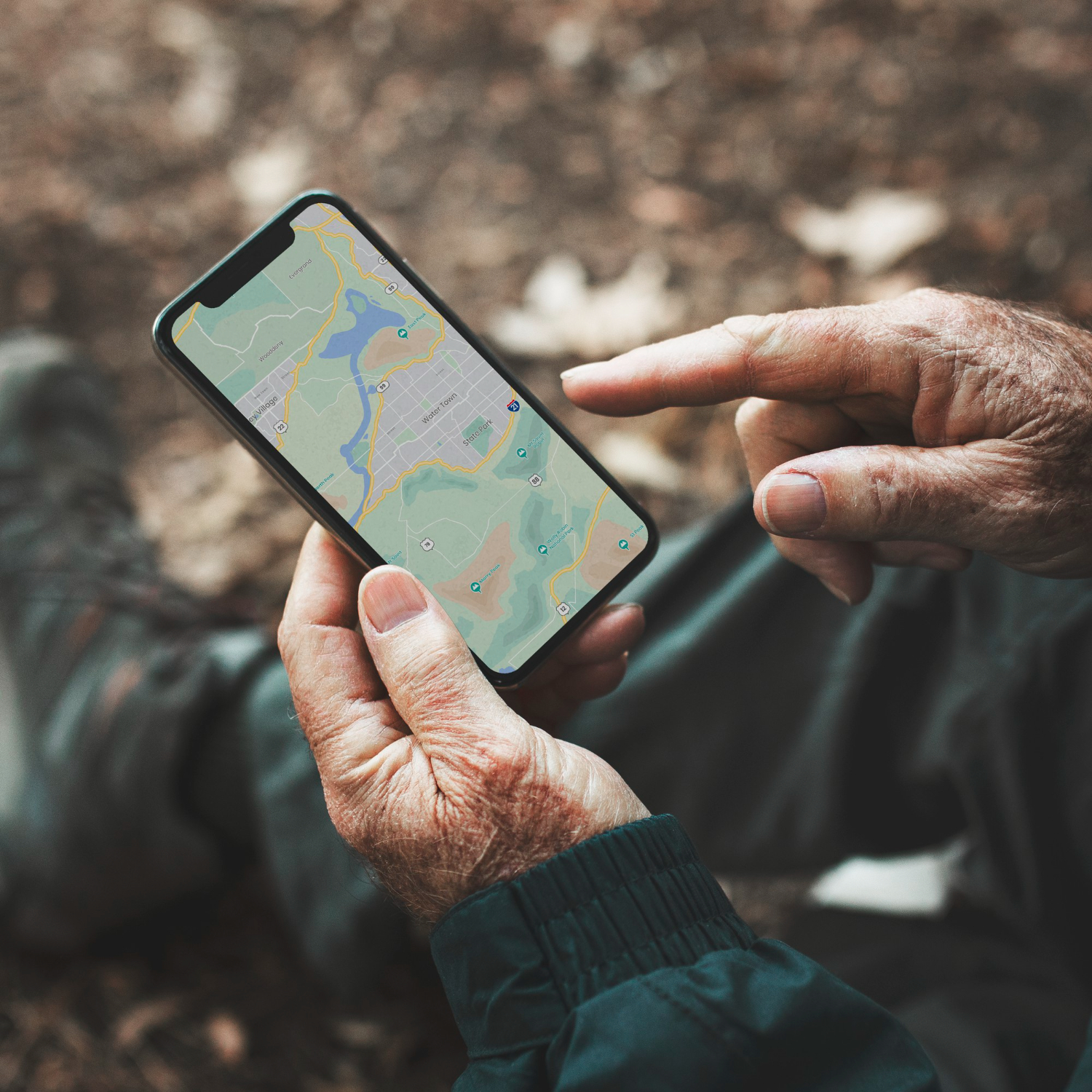
เทคโนโลยี จีพีเอส ถือได้ว่าเป็นเทคโนโลยีที่มีบทบาทในชีวิตประจำวันของเรามาก ๆ ไม่ว่าจะเป็นการใช้ในการเดินทางไปยังสถานที่ต่าง ๆ ช่วยให้เราสามารถเดินทางไปในสถานที่ที่ไม่คุ้นเคยได้ด้วยการใช้แอปพลิเคชันแผนที่ และที่ดีมาก ๆ…
เหตุผลที่ควรติด GPS เพื่อติดตามตำแหน่งของโทรศัพท์ของคุณ
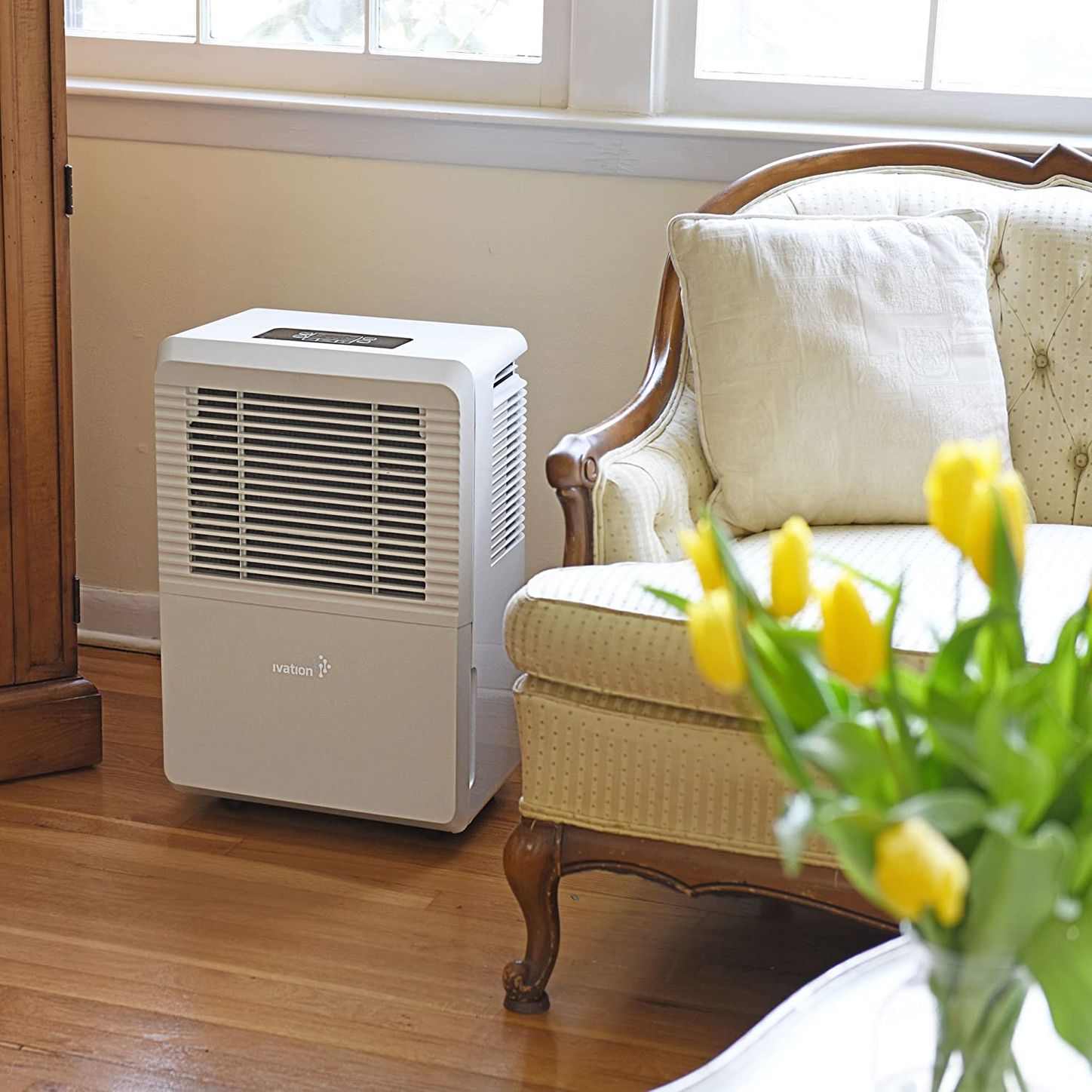
ประเทศไทยมีความชื้นสัมพัทธ์เฉลี่ยอยู่ที่ 70-85% ต่อปี คุณจะเห็นได้ว่ามันเป็นค่าที่ค่อนข้างสูงพอสมควรเลยทีเดียว ดังนั้น จึงเป็นเหตุผลที่ทำให้เกิดเชื้อราได้มากและเป็นสาเหตุของโรคภูมิแพ้ต่างๆ ตามมาด้วย เครื่อง dehumidifier จึงเข้ามามีบทบาทสำคัญถูกใช้ในที่อยู่อาศัยอาคาร…
เครื่อง dehumidifier หลักการทำงานเป็นอย่างไร มีกี่แบบ เหมาะกับใคร
Hot News
โรงไฟฟ้าพลังงานน้ำ ในเครือ gpscgroup
สำหรับใครที่กำลังมองหาแหล่งพลังงานไฟฟ้าจากพลังงานหมุนเวียนวันนี้เรามีอีกหนึ่งรูปแบบพลังงานให้คุณได้ลองเข้ามาทำความรู้จักกับ gpscgroup ซึ่งเราเป็นตัวกลางที่จะทำให้ลูกค้าสามารถเข้าถึงแหล่งพลังงานไฟฟ้าที่มีคุณภาพและเชื่อถือได้โดย gpscgroup มีหลากหลายพลังงานให้ลูกค้าได้ลองเข้ามาทำความรู้จักและเลือกใช้งานโดยเฉพาะโรงไฟฟ้าพลังงานน้ำในเครือของเรามีถึง 3 โรงไฟฟ้าพลังงานน้ำใหญ่ให้ลูกค้าได้ลองเข้ามาทำความรู้จักและดูประสิทธิภาพเผื่อว่าคุณจะกำลังมองหาแหล่งพลังงานไฟฟ้าซึ่งเป็นพลังงานหมุนเวียนที่มีประสิทธิภาพสำหรับโรงงานอุตสาหกรรมหรือแหล่งธุรกิจชั้นนำเอาเป็นว่าถ้าคุณสนใจลองเข้ามาทำความรู้จักกับโรงไฟฟ้าพลังงานน้ำในเครือ gpscgroup กันก่อนได้เลย 3 โรงไฟฟ้าพลังงานน้ำ ที่ดีที่สุด ของ gpscgroup สำหรับใครที่ยังไม่รู้จักโรงไฟฟ้าพลังงานน้ำวันนี้เราจะมาแนะนำ 3 โรงไฟฟ้าพลังงานน้ำที่ดีที่สุดของ gpscgroup ให้ลูกค้าได้รู้จักเผื่อว่ามันจะเป็นอีกหนึ่งความมั่นใจที่ทำให้คุณสามารถเชื่อถือได้ว่าหากคุณเข้ามาเลือกใช้พลังงานไฟฟ้ากับเราจะทำให้คุณได้รับไฟฟ้าได้ตามจำนวนที่ต้องการอย่างแน่นอน โรงไฟฟ้าห้วยเหาะ…
เครื่องร่อนแยกขนาด มีแบบไหนบ้าง ทำงานอย่างไร
เครื่องร่อนแยกขนาดสามารถร่อนอะไรได้บ้าง เครื่องร่อนแยกขนาดเป็นเครื่องจักรที่ทันสมัยใช้ในการร่อนกองวัตถุดิบต่างๆ เช่น ร่อนเม็ดพลาสติก ร่อนแก้ว ร่อนแป้งอุตสาหกรรม ไปจนถึงร่อนปูน/ซีเมนต์ ฯลฯ เครื่องร่อนแยกขนาดมีด้วยกันหลายแบบปัจจุบันนิยมเครื่องร่อนอุตสาหกรรมระบบสั่น เครื่องร่อนระบบสั่นแบบสุญญากาศและเครื่องแยกขนาดระบบสั่น เครื่องร่อนอุตสาหกรรมระบบสั่น เครื่องร่อนอุตสาหกรรมระบบสั่นเป็นเครื่องร่อนแยกขนาดที่มีประสิทธิภาพและมีเอกลักษณ์เฉพาะตัวมีความสามารถในการกรองด้วยตะแกรงแบบละเอียดในอัตราที่รวดเร็ว หลักการทำงานคือการเคลื่อนไหวแบบ 3 มิติผ่านการกรองไวโบรอุตสาหกรรม เครื่องร่อนอุตสาหกรรมที่ดีจะต้องมีความแข็งแรงทนทาน บางเครื่องสามารถสร้างแรงสั่นสะเทือนได้มากถึง 3 เท่าทำให้วัตถุไหลผ่านตะแกรงด้วยความรวดเร็วสูงในอัตราที่สูงกว่าเครื่องร่อนทั่วๆ ไปเครื่องกรองที่ทรงพลังสามารถคัดขนาดได้ถึง…
แนะนำการ์ดจอที่เหมาะสมกับการใช้งาน
ในการทำงานด้วยคอมพิวเตอร์หรือโน๊ตบุ๊คนั้น การเลือกซื้อคอมพิวเตอร์และโน๊ตบุ๊คที่ดีควรเลือกให้สอดคล้องกับการนำมาใช้งานของเรา ยกตัวอย่างเช่น หากเราจำเป็นต้องทำงานด้านกราฟิคที่เน้นในเรื่องการใช้โปรแกรมต่าง ๆ เราควรเลือกซื้อคอมพิวเตอร์หรือโน๊ตบุ๊ครุ่นที่มีสเปคสูง ๆ หรืออาจต้องพิจารณาในเรื่องของการ์ดจอร่วมด้วย โดยตัวการ์ดจอนี้ถือเป็นคีย์หลักในการทำงานของคอมพิวเตอร์และโน๊ตบุ๊คเลยก็ว่าได้ ซึ่งการ์ดจอ (Graphic card) หรือที่เราอาจคุ้นเคยว่า VGA หรือ GPU นั้นจะเป็นตัวที่ทำหน้าที่ในการเพิ่มประสิทธิภาพให้กับการทำงานคอมพิวเตอร์หรือโน๊ตบุคของเรา ซึ่งตัวการ์ดจอนี้ก็มีความแตกต่างกันออกไปตามแต่ละประเภท ดังนั้นนอกจากเราต้องเลือกคอมหรือโน๊ตบุ๊คให้มีสเปคตามการใช้งานแล้ว การเลือกการ์ดจอก็มีความสำคัญไม่แพ้กัน…
เป็นเจ้าของสุขภาพดีแบบง่าย ๆ เพียง Order Food Online From Easy Health
ในช่วงเวลาที่ไม่มีใครอยากออกจากบ้าน การเลือกสั่งอาหารผ่านช่องทางออนไลน์ดูเหมือนจะเป็นตัวเลือกที่ได้รับความนิยมอย่างรวดเร็ว เพราะนอกจากสะดวก ไม่ต้องไปต่อคิวซื้ออาหารด้วยตนเองแล้ว ยังไม่ต้องเสี่ยงรับเชื้อโรคที่มีอยู่มากมายอีกด้วย โดยเฉพาะหากคุณเป็นคนรักสุขภาพที่ชอบดูแลตัวเองให้แข็งแรงเสมอ และสำหรับคนชอบดูแลร่างกายและกำลังมองหาอาหารเพื่อสุขภาพ สามารถเป็นเจ้าของสุขภาพดีได้แค่ปลายนิ้ว เพียง Order Food Online From Easy Health ร้านอาหารเพื่อสุขภาพที่สายสุขภาพน่าจะรู้จักกันเป็นอย่างดี Easy Health ร้านอาหารเพื่อสุขภาพที่คนรักสุขภาพไว้วางใจ แค่ชื่อร้านก็การันตีได้แล้วว่าเป็นร้านที่จัดเตรียมเมนูอัดแน่นด้วยประโยชน์ดี…
ประกันชั้น 1 ราคาถูก กับ เว็บรู้ใจ
เมื่อทุกคนรู้กันดีอยู่แล้วว่าการเลือกซื้อประกันชั้น 1 ราคาถูกถือว่าเป็นรูปแบบประกันภัยรถยนต์ที่ได้รับความนิยมและเป็นที่ชื่นชอบสำหรับผู้เอาประกันอย่างมากดังนั้นจึงไม่ใช่เรื่องแปลกที่วันนี้จะมีคนให้ความสนใจเลยเข้ามาทำความรู้จักกับรูปแบบประกันชั้น 1 ราคาถูกกันเพิ่มมากขึ้นทุกวัน และแน่นอนถ้าคุณเป็นหนึ่งในคนที่สนใจและต้องการเลือกซื้อประกันชั้น 1 ราคาถูกสามารถเข้ามาเลือกซื้อได้ที่เว็บรู้ใจซึ่งเป็นเว็บที่เปิดให้บริการมานานเกี่ยวกับการขายประกันภัยรถยนต์ทำให้คุณมั่นใจได้ว่าทุกบริการทั้งก่อนและหลังการขายจากเว็บรู้ใจนั้นเป็นบริการที่ดีที่สุดช่วยทำให้คุณได้รับรูปแบบความคุ้มครองประกันชั้น 1 ราคาถูกที่สุดและไม่ต้องกังวลเกี่ยวกับปัญหาการเคลมประกันใดๆทั้งสิ้นเพราะรู้ใจเราพร้อมให้ความช่วยเหลือและดูแลในทุกๆขั้นตอน เลือกซื้อประกันชั้น 1 ราคาถูกกับเว็บรู้ใจก่อนใคร สำหรับคนที่กำลังมองหาแหล่งเลือกซื้อประกันชั้น 1 ราคาถูกเราขอแนะนำให้คุณได้ลองเข้ามาทำความรู้จักและเลือกซื้อประกันชั้น 1 ราคาถูกนี้กับเว็บรู้ใจเว็บที่เปิดให้บริการเกี่ยวกับรูปแบบประกันรถยนต์มาเป็นเวลานานแถมมีหลากหลายประเภทประกันรถยนต์ให้คุณได้เลือกซื้อแล้วแต่ความต้องการของผู้เอาประกันเองแน่นอนว่าคุณสามารถเข้ามาทำความรู้จักกับรูปแบบประกันรถยนต์แต่ละประเภทภายในเว็บก่อนที่จะเลือกซื้อได้เพื่อที่จะทำความเข้าใจในรูปแบบความคุ้มครองข้อยกเว้นความคุ้มครองและเงื่อนไขบริการต่างๆเกี่ยวกับประกันรถยนต์แต่ถ้าคุณกำลังมองหารูปแบบประกันชั้น 1 ราคาถูกแน่นอนว่าเข้ามาซื้อประกันกับเว็บรู้ใจสามารถใช้บริการเปรียบเทียบราคาเบี้ยประกันเพื่อเลือกซื้อความคุ้มครองได้ในราคาที่ถูกลงทำให้คุณมั่นใจได้เลยว่าคุณจะมีเงินเหลือเก็บจากการเลือกซื้อประกันรถยนต์กับเว็บรู้ใจอย่างแน่นอน…
ธุรกิจเกี่ยวกับไอทีเติบโตได้ไวจริงหรือ
หากพูดถึงในเรื่องของการทำธุรกิจแล้วนั้นต้องบอกเลยว่าธุรกิจที่มีความเป็นไปได้สูงที่จะได้กำไรได้ไวและสามารถที่จะเติบโตได้ไวนั้นเหมือนจะเป็นในเรื่องของการทำธุรกิจเกี่ยวกับไอทีนั้นเอง เพราะว่าในเรื่องของการทำธุรกิจเกี่ยวกับไอทีนั้นเป็นเรื่องที่สำคัญอย่างมากที่เราเองก็จะต้องอย่ามองข้ามเลย เนื่องจากว่าในส่วนของการทำธุรกิจเกี่ยวกับไอทีนั้นเป็นธุรกิจที่หลายๆคนก็อยากที่จะทำเพราะว่าเป็นธุรกิจที่ลงทุนไปแล้วเราก็ได้กำไรกลับมาได้ง่ายแต่ก็ไม่ใช่เสมอไปทั้งนี้ทั้งนั้นเราจะต้องดูกลุ่มลูกค้าและเทรนอยู่เสมอ เราจะต้องรู้จักที่จะติดตามเทรนอยู่เสมอเพราะยิ่งเราติดตามเทรนได้มากเท่าไหร่ก็จะยิ่งเป็นเรื่องที่ดีมากเท่านั้น การทำธุรกิจเกี่ยวกับไอทีนั้นเราอาจจะไม่ใช่ร้านที่ใหญ่โตในเริ่มแรกแต่เราก็อาจจะไปหาแหล่งรับของจากที่จีนมาขายก่อนก็ได้แต่ทั้งนี้เราจะต้องเลือกของที่คิดว่ามีความเป็นไปได้ที่คนจะใช้หรือมีแนวโน้มว่าจะต้องซื้อกันอย่างแน่นอนก็ควรที่จะเอามาขายเพราะว่ายิ่งเราเอาของมาขายได้ไวหว่าเร็วกว่ามากเท่าไหร่แล้วก็จะยิ่งเป็นเรื่องที่ดีมากเท่านั้น การทำธุรกิจเกี่ยวกับไอทีสามารถที่จะเติบโตได้ไวเราเองจึงควรที่จะต้องใส่ใจและหาข้อมูลอยู่เสมอเพื่อที่จะได้ป้องกันความผิดพลาดที่อาจจะเกิดขึ้นมาได้ เราถ้ามีเงินทุนน้อยเราก็จะต้องยิ่งวางแผนการเงินให้ดีเพราะถ้าหากเราไม่รู้จักที่จะมีการวางแผน ซื้อไปหมด เห็นอะไรสวยก็ซื้อไปหมดเลยอันนี้อาจจะทำให้เราเงินขาดมือได้ด้วย เพราะเราก็ยังไม่รู้ว่าสิ่งที่เราจะนำมาขายนั้นมีคนชอบหรือไม่ชอบอย่างไร เราก็ควรที่จะต้องเลือกแบบละนิดแบบละหน่อยมาลงขายก่อนซึ่งถ้ามีผลตอบรับที่ดีจากลูกค้าแล้วเราก็สามารถที่จะสั่งมาขายใหม่ได้เรื่อยๆแต่เราก็ควรที่จะกะสั่งสินค้ามาขายในแต่ละล็อตให้พอประมาณ ไม่ใช่น้อยเกินไปและมากจนเกินไป ถ้าหากขายไม่ได้และมีเทรนใหม่ๆเข้ามาอีกก็จะทำให้เรานั้นจมทุนได้มากยิ่งขึ้นด้วย เราจึงควรทีจะต้องใส่ใจและให้ความสำคัญกับเรื่องของธุรกิจเกี่ยวกับไอทีให้มากๆเพราะยิ่งเราทำธุรกิจเกี่ยวกับไอทีให้เติบโตได้ไวมากเท่าไหร่เราก็จะได้ลูกค้ามากยิ่งขึ้นแล้วเราก็จะได้กำไรกลับมาที่มากขึ้นกว่าเดิมอีกด้วย…